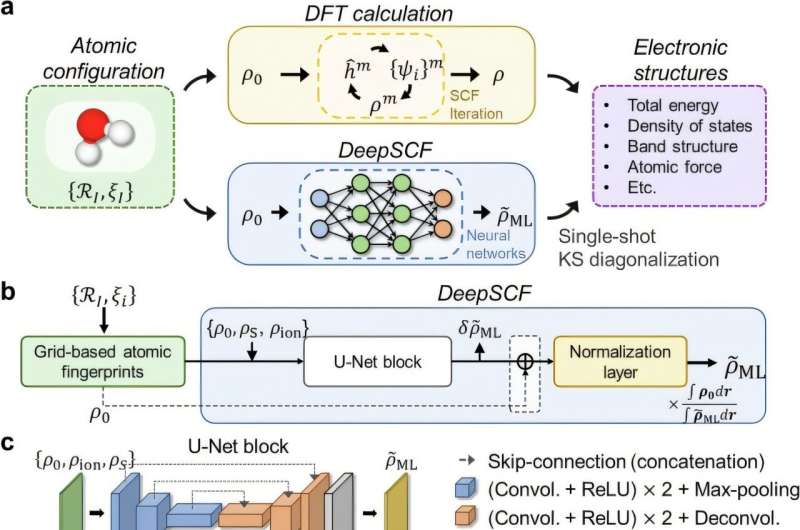
October 30, 2024 by The Korea Advanced Institute of Science and Technology (KAIST)
Collected at: https://phys.org/news/2024-10-ai-method-drastically-shorten-quantum.html
The close relationship between AI and highly complicated scientific computing can be seen in the fact that both the 2024 Nobel Prizes in Physics and Chemistry were awarded to scientists for devising AI for their respective fields of study. KAIST researchers have now succeeded in dramatically shortening the calculation time of highly sophisticated quantum mechanical computer simulations by predicting atomic-level chemical bonding information distributed in 3D space using a novel approach to teach AI.
Professor Yong-Hoon Kim’s team from the School of Electrical Engineering has developed a 3D computer vision artificial neural network-based calculation methodology that bypasses the complex algorithms required for atomic-level quantum mechanical calculations performed using supercomputers to derive the properties of materials.
The density functional theory (DFT) calculations in quantum mechanics using supercomputers have become an essential and standard tool in a wide range of research and development fields, including advanced materials and drug design, as they allow for fast and accurate prediction of quantum properties.
However, in current density functional theory (DFT) calculations, a complex self-consistent field (SCF) process of generating three-dimensional electron densities and solving quantum mechanical equations must be repeated tens to hundreds of times, which limits its application to hundreds or thousands of atoms.
Professor Yong-Hoon Kim’s research team asked whether it would be possible to avoid the self-consistent field process using the artificial intelligence technique that has recently been rapidly developing. As a result, they developed the DeepSCF model to accelerate calculations by learning chemical bond information distributed in three-dimensional space through a neural network algorithm in the field of computer vision.
The research is published in the journal npj Computational Materials.
The research team focused on the fact that according to density functional theory, electron density contains all the quantum mechanical information of electrons, and in addition, the residual electron density, which is the difference between the total electron density and the sum of the electron densities of the constituent atoms, contains chemical bond information, and selected it as a target for machine learning.
Afterwards, the team adopted a data set of organic molecules containing various chemical bond characteristics, and the atomic structures of the molecules included in it were subjected to arbitrary rotations and deformations to further improve the accuracy and generalization performance of the model. Finally, the research team demonstrated the validity and efficiency of the DeepSCF methodology for complex and large systems.
Professor Yong-Hoon Kim, who led this research, said, “We have found a way to correspond quantum mechanical chemical bonding information distributed in three-dimensional space to an artificial neural network. Since quantum mechanical electronic structure calculations are the basis for all-scale material property simulations, we have established the overall basic principles for accelerating material calculations through artificial intelligence.”
More information: Ryong-Gyu Lee et al, Convolutional network learning of self-consistent electron density via grid-projected atomic fingerprints, npj Computational Materials (2024). DOI: 10.1038/s41524-024-01433-0
Journal information: npj Computational Materials
Leave a Reply