OCTOBER 20, 2021 by KeAi Communications
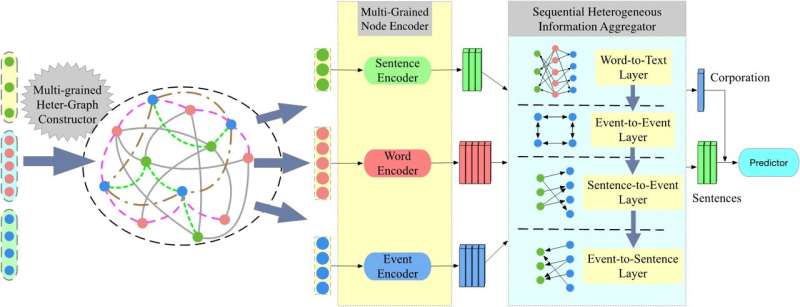
For financial investors, finding ways to effectively predict the behavior of stocks and shares is critical if they want their investments to perform well. There are online sources of information on the factors that drive stock market movements, ranging from news items to financial reports. But developing models that can draw on these various forms of natural language data to create accurate predictions isn’t easy. In fact, for the natural language processing community, it’s a major challenge.
A group of researchers at the Research Center for Social Computing and Information Retrieval at China’s Harbin Institute of Technology have constructed a model that can synthesize these multiple data sources and the various forms of data they contain. Study results, published in the KeAi journal AI Open, show that their model achieves a higher AUC (area under the precision-recall curve) score than existing models.
As author Kai Xiong explains: “Financial texts contain word-level, event-level, and sentence-level information. Simply using a single combination of words, also known as a single semantic unit, isn’t enough to gather all the information you need for an effective prediction model.”
According to co-author Xiao Ding, the Heterogeneous Graph-based Sequential Multi-Grained Information Aggregation Framework (HGM-GIF) they have developed can address this problem.
“To obtain the word-level information, the fine-grained data, our framework uses a stopwords list—in other words, a list of words that should be filtered out when processing the natural language data. To obtain the event information, the medium-grained data, we use an existing openIE tool to extract a series of event triples, comprised of subject, verb and object, from financial text. While to obtain information from the sentences, the coarse-grained data, we split the sentences found in financial text.”
Author Li Du picks up the story: “To model the rich connections between those various sets of data, we use heuristic rules to build connections between words, event triples and sentences. This results in a novel heterogeneous graph neural network that models their interactions.”
In their model, words sequentially interact with text (event triples and sentences) for information selection, event triples interact with event triples for event relationship understanding, sentences interact with event triples for context information supplement, and event triples interact with sentences for information selection. Author Ting Liu adds: “We then pair the results with information about the particular corporation to produce the final stock market prediction.”
The team also conducted studies in which they removed different kinds of information and graph neural network layers from the model to investigate the impact. According to author Bing Qin, these ‘ablation’ studies showed that words, event triples, and sentences are all important for information selection, while each information aggregation layer is important for final stock market prediction.
More information: Kai Xiong et al, Heterogeneous graph knowledge enhanced stock market prediction, AI Open (2021). DOI: 10.1016/j.aiopen.2021.09.001
Provided by KeAi Communications
Collected at: https://techxplore.com/news/2021-10-nlp-stock.html?utm_source=nwletter&utm_medium=email&utm_campaign=daily-nwletter