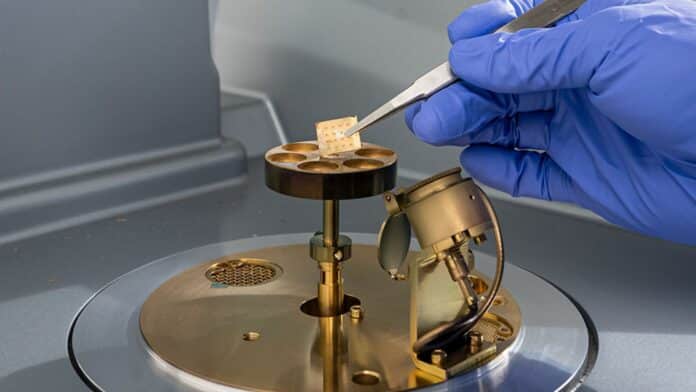
By Jay Kakade 5 Dec, 2024
Collected at: https://www.techexplorist.com/ai-discover-record-breaking-material-film-capacitors/94137/
In a collaborative study of several institutions, researchers and engineers have successfully demonstrated the use of the machine-learning model to accelerate the rate of discovering a perfect material for film capacitors.
This model, feedforward neural networks, searched around 50,000 chemical structures to identify the material with record-breaking performance. The models identified three particularly favorable materials.
Film capacitors are essential compounds in inverters that convert solar and wind generation into alternating current power. This power can then be used in power grids. Given its significance in electric vehicles, power electronics, and aerospace, there is a growing demand for film capacitors.
While batteries use chemical reactions to store and release energy, capacitors use applied electric fields to charge and discharge energy. Film capacitors ensure a quality power supply by preventing ripple currents and ensuring stable and safe operations.
Polymers are considered the best candidates for insulating film capacitors’ materials because they are lightweight and endurance in applied electric fields. However, polymers have limited temperature endurance, and intense heat can degrade them.
Researchers have been looking to uncover the best material to endure highly intense heat. The traditional method involves trial and error, synthesizing materials,s and characterizing their properties. However, this approach is too slow to discover a promising compound from various chemicals.
“Because of the pressing need for better capacitors, this approach is too slow to find promising molecules from the hundreds of thousands of possibilities,” said He Li.
The team developed a machine-learning model known as feedforward neural networks to rev this process. The feedforward neural networks screened through 50,000 for optimal properties that resist high temperatures and electric fields.
“For cost-effective, reliable renewable energy technologies, we need better performing capacitor materials than today. This breakthrough screening technique will help us find these ‘needle-in-a-haystack’ materials,” said Yi Liu.
Researchers separated the three most suitable polymers using a powerful technique called click chemistry. In Berkeley Lab, researchers crafted film capacitors using these polymers and noted their results.
The team found that one of the three polymers displayed a record-high combination of heat resistance, insulating properties, energy density, and efficiency. Additional tests revealed superior material quality and operational stability.
“Our AI analysis quickly identified some key variables in the polymer design details that were predicted to add big improvements in the shielding properties of these polysulfate membranes. As reported in our new Nature Energy study, these earliest machine learning predictors for improving the capacitors are dramatically born out by experiment,” said K. Barry Sharpless.
Journal Reference:
- Li, H., Zheng, H., Yue, T., Xie, Z., Yu, S., Zhou, J., Kapri, T., Wang, Y., Cao, Z., Zhao, H., Kemelbay, A., He, J., Zhang, G., Pieters, P. F., Dailing, E. A., Cappiello, J. R., Salmeron, M., Gu, X., Xu, T., . . . Liu, Y. (2024). Machine learning-accelerated discovery of heat-resistant polysulfates for electrostatic energy storage. Nature Energy, 1-11. DOI: 10.1038/s41560-024-01670-z
Leave a Reply