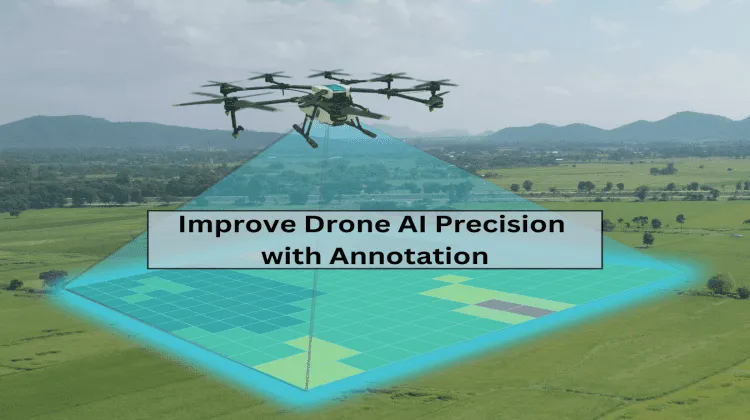
Nick Pegg November 28, 2024
Collected at: https://datafloq.com/read/improving-drone-ai-capabilities-through-visual-data-annotation/
AI-powered drones might be stealing headlines for their futuristic capabilities, but the real power lies in the silent technology that fuels their intelligence, i.e., computer vision and machine learning. From facilitating object detection to crop monitoring and self-navigation, drones rely on training data to “learn” and “understand” their surroundings. Without it, even the smartest drones are flying blind. But how can we ensure AI gets the most reliable data to improve the precision of drones? This is possible with image and video annotation. By accurately labeling the visual data, we can train AI systems to enhance the precision, efficiency, and tracking capabilities of drones. Let’s understand how image and video annotation can elevate drone performance across diverse sectors.
How Computer Vision Works in Drone Technology?
Combining image processing, machine learning, and robotics capabilities, computer vision algorithms enable drones to understand and identify complex scenarios. Equipped with cameras and sensors like LiDAR, RGB, or infrared (depending on their purpose), drones capture images and videos, which are then processed by computer vision algorithms. These algorithms extract important features, patterns, and elements from the visual data to help the drone understand what it’s “looking at.” This enables the drone to perform tasks autonomously, making it more efficient in operations such as surveillance, mapping, and monitoring.
Applications of Machine Learning and Computer Vision in Drones:
Object and obstacle detection
Computer vision algorithms, such as Convolutional Neural Networks (CNNs), facilitate real-time object detection. These models analyze images frame by frame, identifying and classifying objects (like vehicles, humans, or animals) based on pre-learned features. The models are trained on large datasets of labeled images to recognize specific patterns, shapes, textures, and colors associated with different objects. Moreover, they incorporate depth mapping techniques to estimate the distance between the drone and surrounding obstacles, such as buildings or trees, avoiding collisions and ensuring safe navigation during flight.
Self-navigation
By interpreting data from onboard sensors and cameras, drones create real-time maps of their environment, identifying obstacles and dynamically adjusting their flight paths. Algorithms like SLAM (Simultaneous Localization and Mapping) enable drones to localize themselves while mapping unfamiliar environments. Machine learning models allow drones to learn from previous flights, improving navigation efficiency over time. Advanced computer vision algorithms allow drones to utilize pre-defined GPS coordinates to determine departure and destination points and find the best route without manual control.
Remote area monitoring
Drones serve as a vital tool in remote area monitoring by accessing hard-to-reach terrains or hazardous locations. Equipped with computer vision, drones can identify objects, environmental changes, or wildlife in remote regions, making them invaluable for environmental research, disaster response, and wildlife monitoring. Utilizing machine learning capabilities, they can process the collected data to identify patterns or anomalies, such as illegal mining or poaching activities. This real-time analysis allows for swift action, aiding in environmental conservation and disaster management.
Smart city management
Utilizing computer vision and machine learning algorithms, drones can monitor traffic patterns, detect illegal parking, or track environmental changes such as pollution levels to facilitate smart city management. They can analyze real-time data to optimize traffic light timings, reducing congestion and improving commute times. In events or emergencies, drones assist in crowd management by analyzing movement patterns and identifying potential bottlenecks.
Precision mapping
Precision mapping involves creating detailed and accurate representations of geographical areas, and drones are at the forefront of this field. For instance, in agriculture, drones can create highly detailed soil maps by utilizing techniques such as LiDAR scanning and photogrammetry. These soil maps can then be analyzed by machine learning algorithms to provide critical insights into variations in soil health, moisture levels, and nutrient distribution across large farming areas.
Improving Drone Surveillance with Annotation
The accuracy and efficiency of drone AI depend upon the quality of its training data. If the data feeding into the drone’s algorithms is accurately labeled and diverse, it helps computer vision algorithms to efficiently perform tasks like object detection, navigation, and security surveillance. Let’s understand how various image and video labeling techniques can be leveraged to create annotated training data for drone AI, enabling them to handle real-world scenarios.
- Enhancing AI Accuracy to Understand Complex Environments with Multi-Label Annotation
In high-density environments like urban areas, drones must process multiple elements simultaneously-vehicles, pedestrians, infrastructure-all within a single frame. Multi-label annotation allows AI systems to assign multiple tags to different objects in an image or frame, ensuring the drone doesn’t miss any critical details. This approach is particularly effective in complex scenarios where accurate tracking of numerous moving objects is essential, such as in traffic management or monitoring public events.
- Improving Object Detection Capabilities with Fine-Grained Classification
In surveillance, context is everything. Sometimes, subtle differences matter-like distinguishing between a delivery truck and a police vehicle in a crowded space. Fine-grained classification, achieved through detailed annotation, lets drones go beyond just identifying general objects. It’s about training them to see the finer details: Is the construction worker equipped with proper safety gear, or is someone trespassing in a restricted area? By adding layers of understanding to aerial surveillance, fine-grained classification helps drones make more informed decisions that can keep people and property safer.
- Reducing False Positives by Assigning Multiple Attributes to Objects
In environments like airports or secure facilities, it’s crucial to avoid unnecessary alerts that can cause distractions and reduce operational efficiency. Multi-attribute annotation allows drones to analyze objects based on characteristics such as size, speed, and movement patterns. For instance, consider the difference between service vehicles that move in designated areas at predictable speeds and a drone detecting an unauthorized individual running across restricted areas. While both are moving objects, multi-attribute annotation trains the AI to recognize that authorized vehicles operate within defined parameters, whereas a fast-moving individual in an unauthorized zone is likely a security threat.
- Enabling Predictive Surveillance through Behavioral Annotation
By labeling various actions or movements in a video or a frame, training data for drone AI can be created, enabling them to learn and understand patterns. For instance, imagine a drone being used for the surveillance of a parking lot. One car has been circling the area for a suspiciously long time, while another vehicle parked and left immediately. Behavioral annotation tags these actions-such as lingering, erratic driving, or repeated visits, allowing drones to predict potentially dangerous or illegal activities before they escalate.
Real-World Example of How Image & Video Annotation Can Enhance Drone AI’s Capabilities
A US-based technology company provides drone surveillance and security support to businesses across diverse sectors such as agriculture and real estate. To train their object detection algorithms to identify drones’ movements under diverse scenarios and improve their efficiency, they wanted an accurately labeled training dataset. The company outsourced video annotation services to a reliable third-party provider who labeled their aerial footage (captured by other drones) utilizing the bounding box technique. The annotated visual data trained the object detection algorithm to identify drones at different altitudes, in varying lighting conditions, and during all possible flight stages with 30% improved accuracy.
Image Source: SunTec India
Practical Ways to Get Annotated Training Data for Drone AI
To ensure that drones can precisely detect and classify objects in dynamic environments, it is essential to train them on expansive and high-quality data. However, annotating a vast amount of visual data for AI training demands specialized skills, domain expertise, advanced labeling tools, and significant time investment. The two most effective approaches to achieve that can be:
- Invest in Data Annotation Tools and Skilled Labelers
If budget is not a constraint, you can consider hiring skilled data annotators in-house and investing in advanced annotation tools. Initial training can be provided to them to make them aware of your annotation goals, requirements, and specific guidelines. Utilizing diverse automated and manual approaches, these professionals can create high-quality training data for drone AI, meeting your quality standards and expectations.
- Outsource Video and Image Annotation Services to Experts
A more cost-effective approach is to partner with third-party providers for data annotation services. These providers have a dedicated team of skilled annotators and access to a wide range of industry-leading tools to work on large-scale labeling projects with efficiency and precision. Utilizing their domain expertise and years of experience, they can label visual data adhering to the project’s guidelines. This way, you can avoid making significant infrastructure investments and save time to focus on other business aspects.
Key Takeaway
As AI-powered drones continue to reshape industries, the importance of precise image and video annotation cannot be overstated. It’s not just about making drones smarter-it’s about unlocking new levels of accuracy, safety, and autonomy in real-world applications. By refining the way we annotate visual data, we set the stage for a future where drones perform complex tasks with precision and minimal human intervention.
Leave a Reply