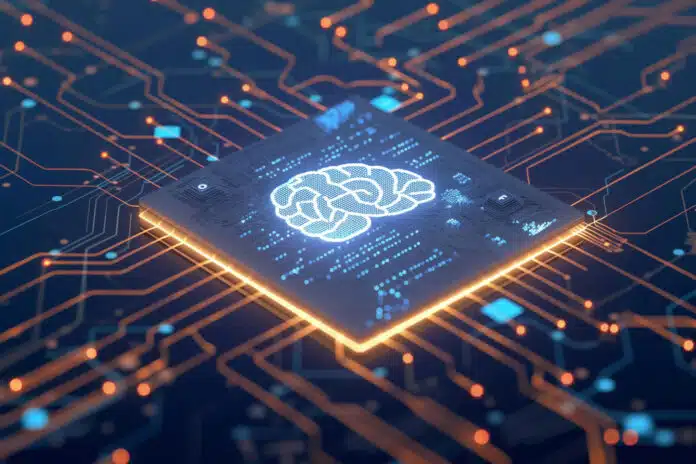
By Ashwini Sakharkar 25 Oct, 2024
Collected at: https://www.techexplorist.com/new-machine-learning-model-predicts-dielectric-function-materials/91586/
Cutting-edge researchers Tomohito Amano and Shinji Tsuneyuki at the University of Tokyo, alongside Tamio Yamazaki from CURIE (JSR-UTokyo Collaboration Hub), have unveiled a machine learning model that revolutionizes our approach to predicting the dielectric function of materials – moving beyond traditional first-principles calculations.
This critical function gauges the polarization of negative and positive charges within materials, forming the core concept behind dielectric materials.
With this innovative model, we can achieve rapid and precise predictions of dielectric functions, paving the way for the creation of next-generation dielectric materials essential for transformative technologies, including the anticipated advancements in 6G network.
While not as widely recognized as semiconductors, dielectric materials hold immense promise for enhancing modern electronic systems. These materials offer a unique characteristic: they do not conduct electricity well but are not insulators either. When subjected to an electric field, positive charges within the material are drawn toward the field, while negative charges shift in the opposite direction, leading to dielectric polarization.
This polarization is quantified by the dielectric function, a critical measure of its strength. Despite its significance, calculating the dielectric function generally requires complex first-principles approaches rooted in quantum mechanics, making it a time-consuming and resource-intensive endeavor.
“The study of dielectrics is important both for fundamental and applied science,” says Amano, the first author. “On the fundamental side, dielectrics can help elucidate the microscopic origin of how materials respond to electric fields. On the applied side, low-dielectric polymer materials for high-speed communication have garnered attention recently.”

Researchers have successfully developed an innovative machine-learning model designed to tackle significant challenges in materials science. By generating training data through first-principle calculations of the electronic states of various materials, they’ve taken a groundbreaking approach.
Instead of relying on conventional calculations focused on individual molecules, this model emphasizes the chemical bonds between atoms. Its accuracy was rigorously tested against empirical data from simple molecules like methanol and ethanol.
This model not only describes the electronic states of a variety of materials with nearly the same accuracy as traditional methods, but it also does so while dramatically reducing computational demands. Its capability for large-scale and long-term simulations opens new doors, enabling researchers to investigate the macroscopic origins of dielectric properties in complex molecular systems—a feat previously hindered by computational costs.
Despite these impressive achievements, Amano is already envisioning future possibilities.
“In this work, we investigated the dielectric properties of simple molecules, but application to more complex molecules, including polymers, is yet to be done. So, we are also planning to construct a universal neural network that can be used in industry.”
Journal reference:
- Tomohito Amano, Tamio Yamazaki, and Shinji Tsuneyuki. Chemical bond based machine learning model for dipole moment: Application to dielectric properties of liquid methanol and ethanol. Physical Review B, 2024; DOI: 10.1103/PhysRevB.110.165159
Leave a Reply