
August 21, 2024 by Journal of Remote Sensing
Collected at: https://phys.org/news/2024-08-plows-pixels-comprehensive-rice-satellite.html
While satellite technology might seem distant and space-bound, its benefits can be seen everywhere.
Scientists from Sun Yat-sen University and their colleagues have developed an innovative framework that aligns advanced satellite data with rice growth stages to bolster paddy rice mapping accuracy. These results are expected to push the boundaries of mapping precision and contribute to global food security efforts.
Their findings were published in the Journal of Remote Sensing on July 8.
Rice is a staple grain in many countries and constitutes a significant share of the global food supply. Accurate paddy rice mapping is essential for coordinating agricultural production, ensuring food security and managing cultivation. Regions in the tropics and subtropics, such as Guangdong, China, are major rice producers. Mapping rice paddies in these areas, however, remains a challenge.
“There are two main difficulties in tropical and subtropical paddy rice mapping: the lack of high-quality optical images and differences in paddy rice planting times,” said Qian Shi, a professor at the School of Geography and Planning at Sun Yat-sen University and lead author of this study.
Tropical and subtropical regions often experience cloud cover, which obscures land surface information on satellite images. Additionally, varying climate conditions and planting habits mean that rice planting times differ, complicating accurate mapping.
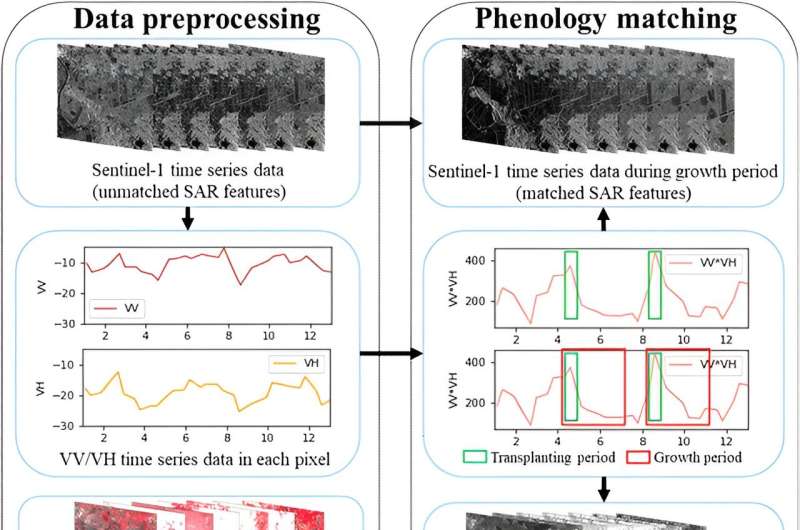
To overcome these obstacles, the team developed a new mapping framework using phenology matching. This technique involves aligning the growth stages of rice plants with data from Sentinel-1 and Sentinel-2 satellites to improve mapping accuracy in Guangdong.
Leveraging Sentinel-1’s ability to penetrate cloud cover, the researchers identified rice growth periods, or phenological periods, and gathered radar and optical data features specific to these periods from both satellites. These features were then fed into machine learning classifiers, or algorithms that categorize data.
“By matching features derived from Sentinel-1 and Sentinel-2 to specific growth stages, our classifiers significantly enhanced mapping accuracy compared to conventional methods,” Shi said.
This multi-source data approach indicated a significant improvement in accuracy, ranging from 6.44% to 16.10%. These advancements were further validated through regression analysis that aligned mapped areas with statistical data.
This study also shed light on critical factors influencing rice phenology and spatial distribution in Guangdong. Thermal conditions, particularly cold severity during growth stages, emerged as primary determinants affecting rice development. The interplay of human and natural factors, including land slope and minimum temperature, also significantly influenced spatial rice patterns.
These findings provide a deeper understanding of rice ecosystem dynamics, offering valuable insights for formulating agricultural policies and underscoring the importance of integrating cutting-edge science with on-the-ground agriculture to optimize production and sustainability.
“We aim to apply this methodology to other tropical and subtropical regions, further refining accuracy and expanding insights into global rice production dynamics,” Shi said.
More information: Sun, L. et al. Paddy Rice Mapping Based on Phenology Matching and Cultivation Pattern Analysis Combining Multi-Source Data in Guangdong, China, Journal of Remote Sensing (2024). DOI: 10.34133/remotesensing.0152
Leave a Reply