
JULY 15, 2024 by JooHyeon Heo, Ulsan National Institute of Science and Technology
Collected at: https://phys.org/news/2024-07-team-satellite-machine-typhoon-intensity.html
Amidst the challenges posed by climate change in predicting typhoons, a team of researchers has developed a technology that leverages real-time satellite data and deep learning capabilities to predict typhoons with greater precision.
Led by Professor Jungho Im in the Department of Civil, Urban, Earth and Environmental Engineering at UNIST, the research team has unveiled a deep learning-based prediction model that combines geostationary weather satellite data and numerical model data in real-time.
The findings have been published in GIScience & Remote Sensing and iScience in March and June 2024, respectively.
The Hybrid-Convolutional Neural Networks (Hybrid-CNN) model, proposed by the research team effectively combines satellite-based spatial characteristics and numerical prediction model outputs to objectively and accurately forecast tropical cyclone (TC) intensity with lead times of 24, 48, and 72 hours. The Hybrid-CNN model demonstrates a significant reduction in uncertainty associated with numerical models, thereby enabling more accurate typhoon forecasting.
The traditional method of observing typhoons relies heavily on geostationary satellite data analyzed by forecasters; however, this approach is hindered by the need for lengthy analysis times and the uncertainty inherent in numerical models. In contrast, the Hybrid-CNN model significantly reduces uncertainty associated with numerical models, thereby enabling more accurate typhoon forecasting.
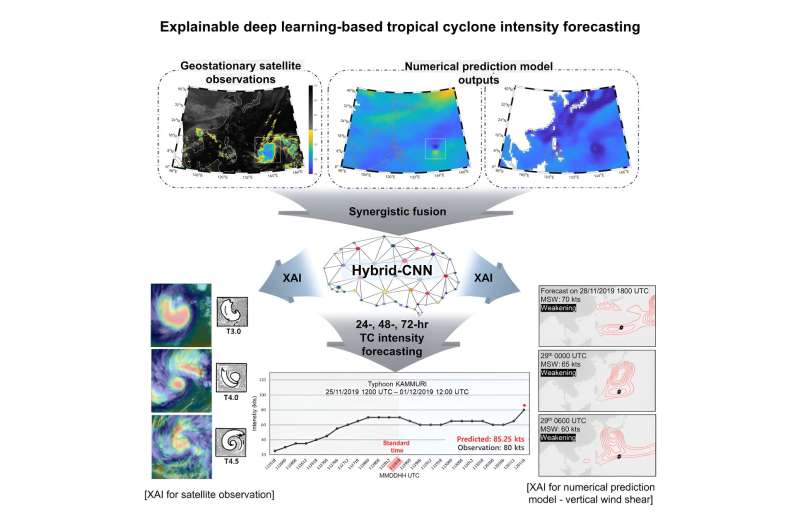
The research team employed a transfer learning model to estimate TC intensity using satellite data from the Communication, Ocean, and Meteorological Satellite (COMS) launched in 2010 and the GEO-KOMPSAT-2A (GK2A) launched in 2019. The AI visualized and quantitatively analyzed the automatic typhoon intensity estimation process to increase the accuracy of typhoon forecasts.
Environmental factors affecting changes in TC intensity can be objectively extracted and applied to the field forecast system. It is expected that this technology will provide significant assistance in disaster preparedness and damage prevention by providing quick and accurate typhoon information to forecasters.
Professor Im stated, “Our deep learning-based typhoon prediction framework will enable forecasters to develop quick and effective measures by providing more accurate prediction information.”
More information: Minki Choo et al, Bridging satellite missions: deep transfer learning for enhanced tropical cyclone intensity estimation, GIScience & Remote Sensing (2024). DOI: 10.1080/15481603.2024.2325720
Juhyun Lee et al, Enhancing tropical cyclone intensity forecasting with explainable deep learning integrating satellite observations and numerical model outputs, iScience (2024). DOI: 10.1016/j.isci.2024.109905
Journal information: iScience
Leave a Reply