by Pam Baker, Contributing Writer on October 17, 2022
Here is a quick look at things you can do to help govern and manage your data in the most practical sense.
If you believe the marketing hype, you’d think data management and governance is a snap. An easy peasey, automated to the hilt, set-it and forget-it, little cleanup task on the prepping end of the serious work: data analysis.
But today it’s more like mapping a mine field while trying not to step on one of the many camouflaged dangers. If you mess this part up, the aftermath will be even messier.
To put it simply: If the data is wrong or incomplete, the analysis will rank somewhere between useless and dangerous. If the data slips through the cracks unnoticed, your company could be at risk of hefty fines and penalties.
Let’s skip the hype then and get down to what works best in terms of practices and processes. Here is a quick look at 10 things you can do to help you govern and manage your data in the most practical sense.
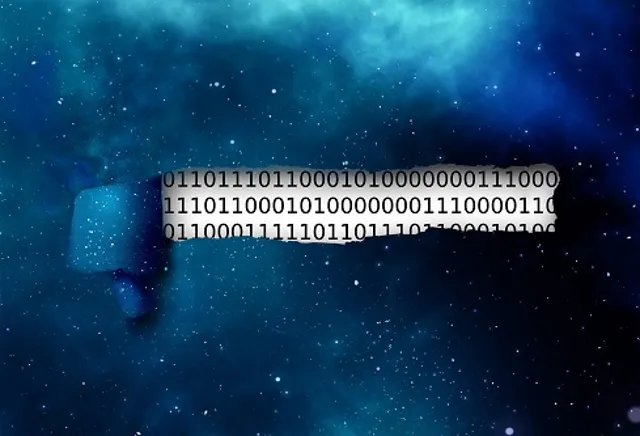
1. Check for Hidden Constraints
There is a natural tendency to consider work constraints but overlook everything else.
“We tend to focus on all of the facets of the work: data ownership, access, security, quality, and so on,” says David Allen, Senior Director of Developer Relations at Neo4j, a producer of a graph database management system. “However, all those things are constrained by the company context they reside in such as data owners who are organizational actors with incentives, pressures, challenges, limitations, and so forth.”
So where else should you look to find constraints on your efforts to manage and govern data?
“In short, pay some, but not too much attention to frameworks and technologies — and never lose sight of the human and organizational element. The practitioner’s job is to do the best they can within a real context, and that almost always looks different than what the textbooks say,” Allen adds.
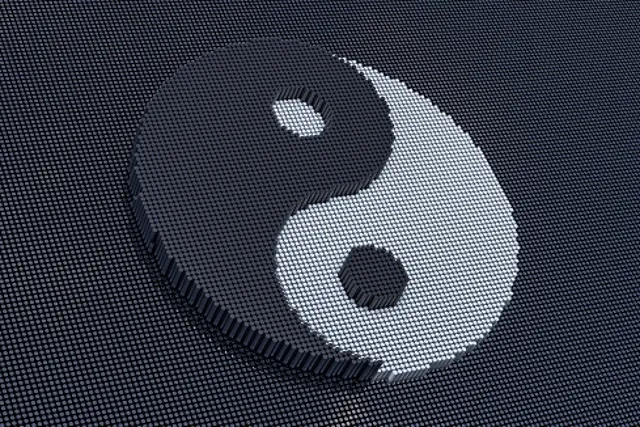
2. Balance the Conflicts
Managing and governing data is rarely a straightforward, unencumbered exercise. It’s usually a mesh of entanglements built of conflicts within and between demands on the business.
“Consumers are simultaneously requesting personalization and privacy, and that’s why businesses are now placing much more value in their own customer data,” says Keyvan Mohajer, CEO of SoundHound, an audio and speech recognition company that develops speech recognition, natural language understanding, sound recognition and search technologies. “First-party data allows brands to create great experiences, but it also puts them in control when it comes to data transparency and privacy.”
Data management and governance becomes much trickier when you lose full control of the data.
“Brands looking to use voice AI are becoming increasingly aware of the risk of handing this data control over to Big Tech voice assistant providers. Having an intermediary not only obstructs a business’ view of valuable user feedback, but it also prevents them from reassuring customers about what their data is used for — and allowing them to opt out,” Mohajer adds.
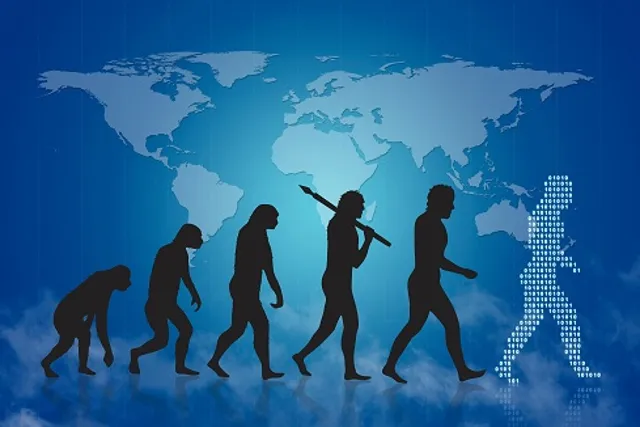
3. Track Data Lineage
Given deep fake attacks and increasing regulatory demands, it’s better to know the origin and the trail for every data set, if not every data point. Without a clear and uncorrupted data trail, you’ll never know whether the data is trustworthy — and neither will auditors, cybersecurity pros, or regulators.
“Less than one third of companies are able to trace their data to the source and ensure that it’s visible to only the authorized parties. At scale this requires ‘guardrails,’ basically reinforcement mechanisms, to combat and prevent regulatory lapses, while still enabling you to use AI to make workflows more efficient,” says Seth Dobrin, IBM’s Global Chief AI Officer.
“These are not insignificant challenges and solving them requires five key technological building blocks to help simplify how we integrate and improve data management and governance: AI-augmented data cataloging, automated metadata generation, automated governance, data virtualization, and reporting and auditing,” he adds.
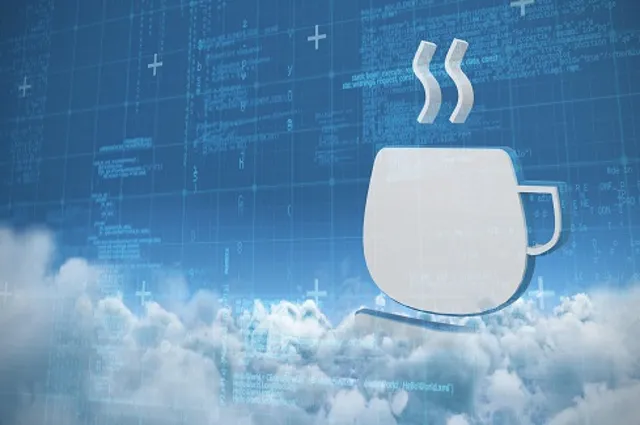
4. Consider a ‘Product Management’ Approach
Organizing data into safe and servable portions per domain use can be a practical way to managing it well.
“Data management is increasingly becoming more of a ‘product management’ practice – curated data sets, built from a number of data sources from across application and business areas become data domains that benefit from the formal requirements gathering, roadmap planning, quality assurance, build automation, and ongoing change management associated with more traditional product development practices,” says James Fairweather, Chief Innovation Officer of Pitney Bowes, a 100+ year old, global shipping and mailing company.
“For example, Pitney Bowes has begun building data domains using concepts associated with data fabric and data virtualization to provide well curated data products for use in analytics, data science modeling, and reporting.” Fairweather says his company uses “tools like SelectStar for data governance, and MonteCarlo to detect anomalies by improving data observability in our pipelines.”
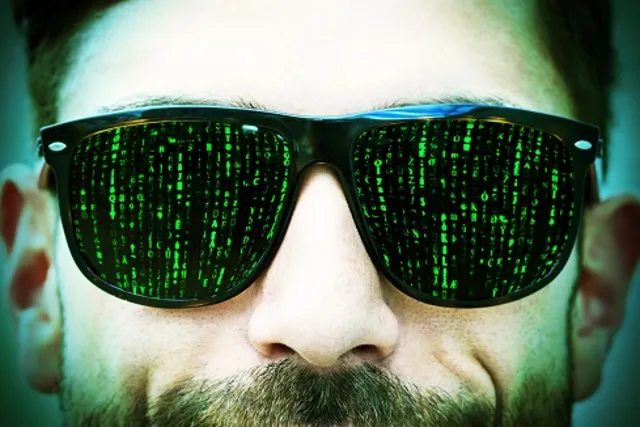
5. Know Thy Data Extremely Well
Yes, data is huge and getting bigger. Yes, it’s pouring in from an ever-growing number of sources. Even so, you must understand it well and truly know what information your company has.
“The best thing corporations can do to manage and govern their data is to intimately know their data,” says Chida Sadayappan, Cloud AI/ML Offering Leader, at Deloitte Consulting. “Understanding data creation, processing, consumption, and retention will help them find appropriate tools and processes to manage and govern their data well.”
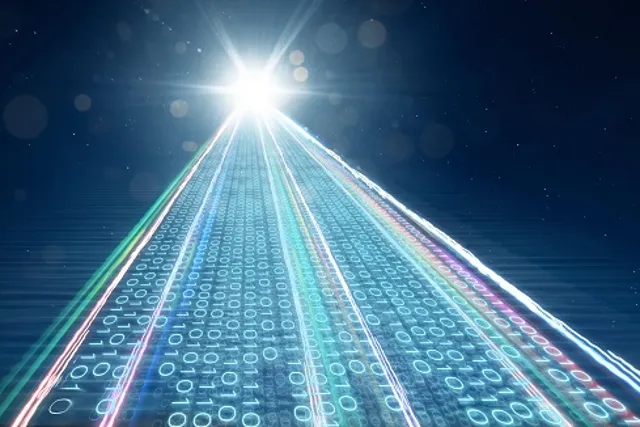
6. Don’t Forget Data Coming Out the Other Side
Companies tend to think of managing data to be ingested and analyzed. But data coming out of the analysis also must be managed, governed and its lineage clearly documented. In other words, make sure you’re managing ALL the data — not just some of it. Unfortunately, that can be quite the challenge.
“Make sure you are taking the time to regularly engage with and understand exactly how your users are currently accessing and utilizing your data,” says Christopher Goranson, service professor at Carnegie Mellon University’s Heinz College. “Understand what they do with the data once they access it — do they aggregate it further? Do they combine it with other datasets? Can they understand what the data represents, and any limitations based on your existing documentation? If your organization provides publicly accessible datasets, how are those used? What questions are they trying to answer?”
“These can often be clues you can use to improve the value of the data you manage to your organization,” Goranson explains.
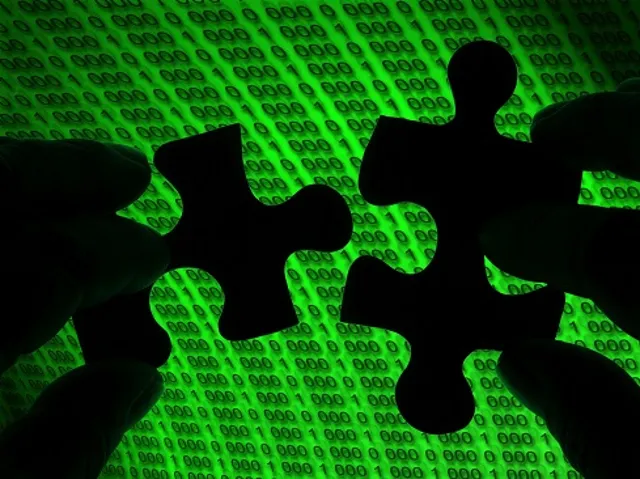
7. Connect the Fragments
Complying with data privacy regulations can break chains of knowledge needed to resolve pressing issues. Consider using technologies that can protect privacy without fragmenting shared data chains needed for collective wins.
“A fundamental issue in data governance is the fragmented nature of data across multiple silos — both internally across borders and externally between firms,” says Michael Hughes, Chief Business Officer at Duality Technologies, a provider of Privacy Enhancing Technologies (PETs). “This creates a challenge for enterprises that need to share and collaborate on this data to derive insights,”
“Banks, for example, rely on collaboration in the fight against fraud, cybercrimes, and money laundering because data exists across providers and jurisdictions. Healthcare research also depends on the sharing of clinical and genomic data to advance treatments. The problem is they can only share data if they can preserve privacy and confidentiality, while maintaining compliance in an increasingly complex regulatory environment and many existing approaches fall short,” Hughes adds.
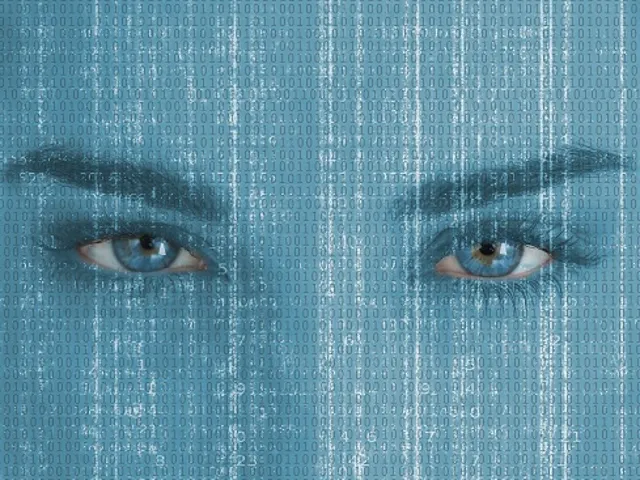
8. Always Name the Problem
As the adage goes, you can’t manage it unless you can manage it. However, you can’t measure it either, unless you can name it. In other words, to err is to be vague. To name it is to define it.
“The easy parts of the equation are financing the governance process and the creation of data management policies,” says Stefan Thorpe, Chief Engineering Officer at Cherre, a real estate data and analytics platform based in New York. “The real challenge comes from enforcing the data management policies, especially when the enterprise is relatively complex in structure. Even simple tasks such as defining and monitoring key performance metrics can be complex when the processes are not well-defined.”
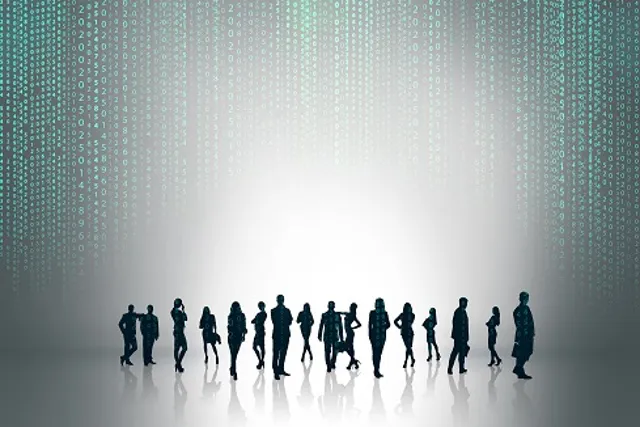
9. Remove the Blinders, Bring On More Eyes
AI can do a lot but it can’t outright replace human workers. At least not yet.
“Data governance is essential to any organization’s data blueprint,” says Manish Sood, Founder and CTO of Reltio, a master data management (MDM) platform. “One of the ways to ensure better governance is by finding ways to put data into the hands of more users but doing so with processes that scale with an organization and create that alignment across teams. It’s simple: the more eyeballs on the data, the better the quality and the more thorough the governance. Or put in even simpler terms, you don’t fix what you can’t see.”
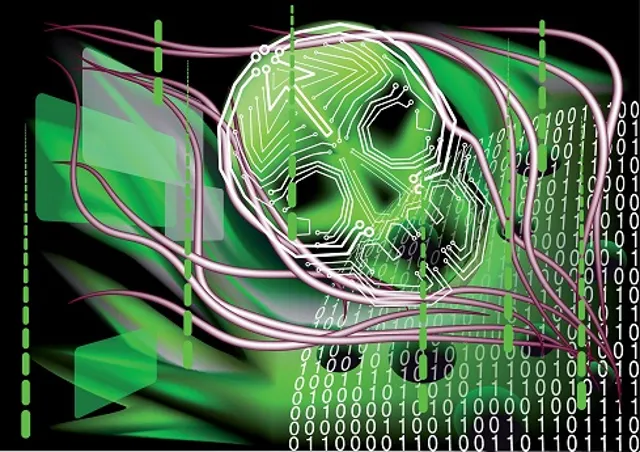
10. Send More Data to the Morgue
Ok, not to the morgue exactly, but certainly to cheaper cold storage. In other words, data is hot until it’s not and there’s no reason to keep it in a warmer when its fine chilled.
“Be aggressive in culling data that you don’t need. Also, minimize the amount of data that is stored in expensive ‘hot’ or ‘warm’ storage. Kick things that you need to keep to cheap ‘cold’ storage as soon as you can,” says Matt Shea, Head of Federal at MixMode AI, an AI powered cybersecurity platform.
Collected at: https://www.informationweek.com/big-data/10-actionable-tips-for-managing-governing-data?slide=1
Leave a Reply