APRIL 22, 2022 by Chinese Academy of Sciences
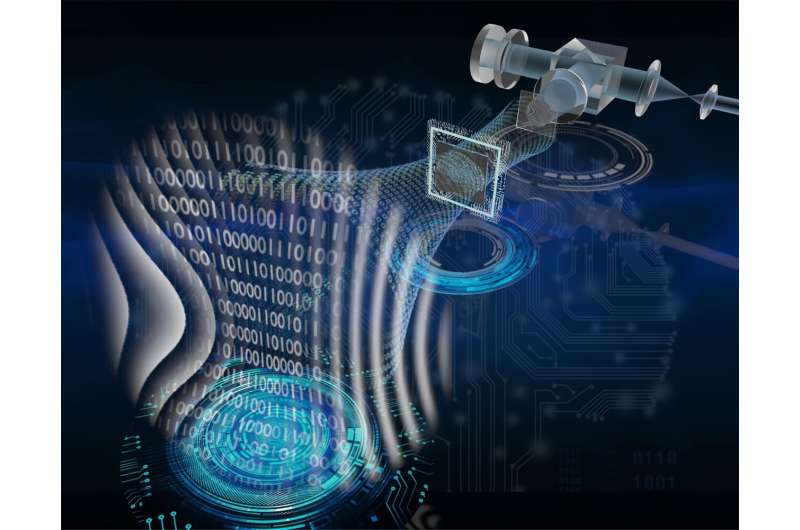
Deep learning is currently prompting increasing research interests and leading to a paradigm shift from physics-based modeling to data-driven learning in the field of optical metrology. Scientists in China and Singapore published a review article entitled “Deep learning in optical metrology: a review” in Light: Science & Applications. They provide a comprehensive review of deep learning in various optical metrology tasks, revealing that problem-specific deep-learning methods, in most cases, considerably outperform their physical model-based predecessors.
Optical metrology is the science and technology of making measurements with use of light as standards or information carriers. Although optical metrology is a rapidly growing area, it is not a new discipline. The development of physical sciences has been driven from the very beginning by optical metrology techniques. In return, optical metrology has been revolutionized by the invention of the laser, charged coupled device (CCD), and computer, developing into a broad and interdisciplinary field relating to diverse disciplines such as photomechanics, optical engineering, computer vision, and computational imaging.
Recently, deep learning (DL), a subfield of machine learning, has been gaining extensive attention for its extensive applications and tremendous successes in computer vision, natural language processing, and computer-aided diagnosis. Meanwhile, tech giants Google, Facebook, Microsoft, Apple, and Amazon have ignited the “art” of data manipulation and developed easy-to-use, open-source deep learning frameworks. Deep learning has left the halls of academia very quickly and is ready to reshape an array of companies across multiple industries. In light of the great success of deep learning in these related fields, researchers in optical metrology were unable to hold back their curiosities with regard to adopting this technology to further push the limits of optical metrology and provide new solutions in order to meet the upcoming challenges in the perpetual pursuit of higher accuracy, sensitivity, repeatability, efficiency, speed, and robustness.
In a recent review paper published in Light Science & Application, the research teams led by Professor Chao Zuo from Smart Computational Imaging (SCI) Laboratory, Nanjing University of Science and Technology, China, and Professor Kemao Qian from the School of Computer Science and Engineering, Nanyang Technological University, Singapore, presented an overview of the current status and the latest progress of applying DL in optical metrology. In this review, they systematically summarized classical techniques and image processing algorithms in optical metrology, and discussed the technical advantages of using DL in optical metrology tasks by interpreting the concept as an optimization problem. Then a comprehensive review of specific applications of DL in various optical metrology tasks was provided. The challenges and future directions of DL techniques in the field of optical metrology were also pointed out and envisioned.
Optical metrology methods often form images (e.g., fringe/speckle patterns) for processing. They can provide full-field measurements at scales ranging from millimeters to nanometers with high speed, sensitivity, resolution, and accuracy. In general, the task of optical metrology is to obtain the desired sample parameter from the observed images, which is a typical inverse problem associated with many challenging problems, such as model mismatch, error accumulation, and ill-posedness. In the fields of computer vision and computational imaging, the classical approach for solving an ill-posed inverse problem is regularization, which reformulates the original problem into a well-posed one by imposing prior assumptions about the solution.
In contrast, in optical metrology, due to the fact that the optical measurements are frequently carried out in a highly controlled environment, researchers prefer to reformulate the original ill-posed problem into a well-posed and adequately stable regression problem by actively controlling the image acquisition process. However, for many challenging applications, harsh operating conditions may make such active strategies a luxurious or even unreasonable request. Under such conditions, deep learning is particularly advantageous for solving those optical metrology problems because the active strategies are shifted from the actual measurement stage to the preparation (network training) stage, and the “reconstruction algorithm” can be directly learned from the experimental data. If the training data is collected under the environment that reproduces the real experimental conditions, and the amount of data is sufficient, the trained model should reflect the reality more precisely and comprehensively, and is expected to produce better reconstruction results than conventional physics-model-based approaches.
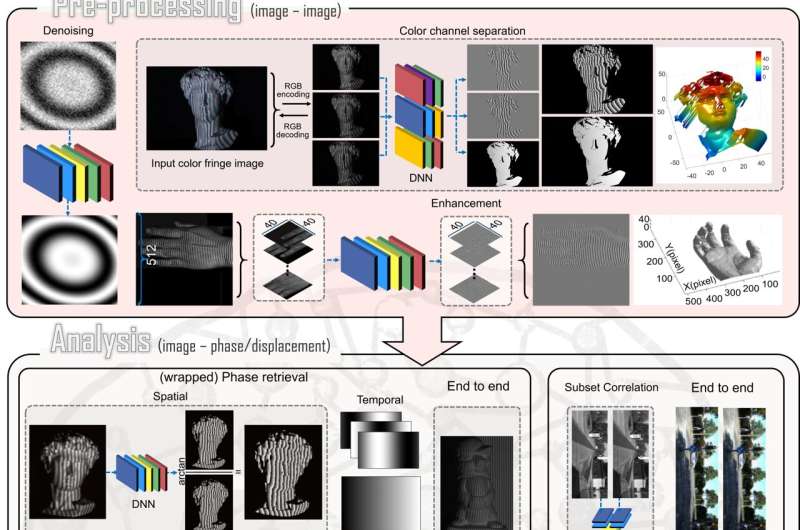
Owing to above mentioned advantages, DL has been gaining increasing attention in optical metrology, revolutionizing the concept of optical metrology and reinventing almost all the fundamental tasks of digital image processing in optical metrology. Deep learning has gradually “penetrated” into almost all aspects of optical metrology, showing promising performance and great potential in fringe analysis, phase retrieval, phase unwrapping, etc.
Nevertheless, the authors pointed out that deep learning is still at the early stage of development for its applications in optical metrology and significant challenges do remain in this field. As an indispensable tool in industrial inspection, medical diagnosis, and scientific research, it is crucial to ensure the results obtained by optical metrology methods are reliable, repeatable, and traceable. However, DL is often been regarded as “black boxes” with no theoretical groundwork to clearly explain why a particular network structure is effective in a given task or not, which may cause severe consequences. In addition, DL learns and extracts the “common” features from the training samples, but this may lead to unsatisfactory results when facing “rare samples” since the information cannot be “born out of nothing.”
“The progress of science comes from the continuous exploration to solve the unknown.” The insights demonstrating possible routes for the further development of DL in optical metrology were envisioned:
- Leveraging more emerging technologies of DL to optical metrology could promote and accelerate the recognition and acceptance of DL in more applications.
- Combining Bayesian statistics with DL to obtain quantitative uncertainty estimates allows to assess when DL yields unreliable predictions.
- A synergy of the physics-based models describing a priori knowledge and data-driven models learning a regularization from the experimental data is expected to provide more physically plausible solutions to optical metrology.
“Will deep learning replace the role of traditional technologies within the field of optical metrology for the years to come? “It is clear no one can predict the future, but we can engage it,” Zuo et al. said. “If you are still an ‘outsider’ or new to this field. We encourage you to try it out! It is easy, and often works.”
More information: Chao Zuo et al, Deep learning in optical metrology: a review, Light: Science & Applications (2022). DOI: 10.1038/s41377-022-00714-x
Journal information:Light: Science & Applications
Provided by Chinese Academy of Sciences