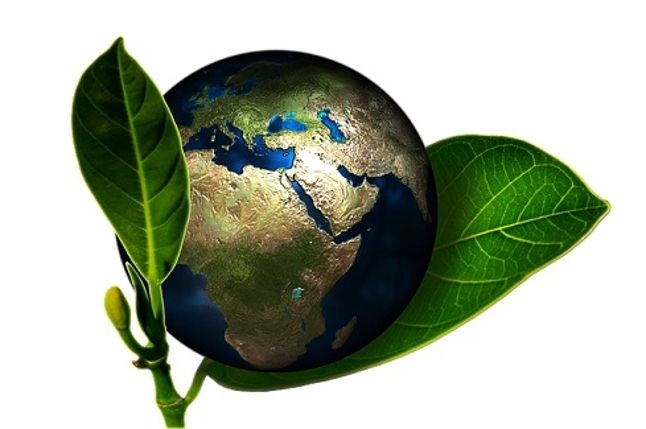
Simon Mingay, Research Vice President, Gartner, Inc., August 09, 2021
Collected at: https://www.informationweek.com/digital-business/9-ways-to-reduce-the-environmental-impact-of-data?_mc=NL_IWK_EDT_IWK_bigdata_20210809&cid=NL_IWK_EDT_IWK_bigdata_20210809&elq_mid=105509&elq_cid=27653255
IT leaders can reduce the environmental impact of their data by considering a set of data sustainability principles.
Digital business has a carbon footprint. While there is a significant focus in the IT industry on the environmental footprint of data centers and cloud services, there isn’t so much on the data itself. Data storage requirements are growing at approximately 50% annually, according to Gartner research — a statistic that cannot be overlooked.
Despite ongoing improvements in data center, network and storage system energy efficiency, the greenhouse gas (GHG)/carbon emissions and environmental footprint of storage will become increasingly significant in terms of the overall footprint of IT, and potentially, of the enterprise itself.
Sustainability touches every aspect of an enterprise’s activities, from the strategy and business model, to product/service design and use/consumption, all aspects of business operations, supply chain and end-of-life recovery or disposal. In fact, nearly half of CEOs reported that climate change mitigation is having a significant impact on their business, IT leaders should apply several principles when developing or reviewing their data strategy and architectures:
Baseline the GHG or carbon footprint of your data, and apply the principle of materiality.https://08f230099fb8da3d1fa273dc503c43e3.safeframe.googlesyndication.com/safeframe/1-0-38/html/container.html
Materiality is a critical principle to any sustainability strategy or program. Using materiality to guide the sustainability strategy means focusing on those things that first are significant in terms of the enterprise’s impact on the environment, society, and second are “important” to the enterprise’s stakeholders.
To establish whether data is material, baseline the energy and GHG footprint of the storage systems, associated supporting network and data center infrastructure. If data is not material, then focus on areas that are. Extend existing data classification approaches to include the materiality perspective, and capture GHG footprint of data as part of the organization’s metadata management process.
Be relentless in removing data that is no longer required, or is of low value.
There’s no need to be a data hoarder without good reason. The mindset of keeping things “just in case” will cause high environmental and financial costs down the line. For this reason, it’s imperative that IT leaders enforce rigorous data life cycle policies, filtering out data that does not contain value. The data that has high value is focused on solving specific problems aligned to business strategy and objectives. This goes hand in hand with refining data close to the source in time and space.
Ensure the quality of data close to the point of origin.
Poor data quality costs organizations an average of $12.9 million dollars per year, according to Gartner. Proper data and analytics governance practices include a focus on the quality of data and the assurance that it is fit to support the business process in which it is consumed. Failure to prevent data quality issues or immediately address them as they occur generally leads to added processing steps downstream. Collaborate with business stakeholders to establish effective and scalable data quality programs aimed at driving desired and sustainable business outcomes.
Host data in energy-efficient, low-carbon data centers.
Choice of data center and/or data center service provider will have a big impact on the overall energy, GHG and water footprint.
Data center energy efficiency can vary substantially. For example, modern hyperscale data centers are usually incredibly energy efficient, with power usage effectiveness (PUE) below 1.2. In contrast, the average enterprise data center is usually relatively inefficient. The energy efficiency of the hosting site must be an explicit part of an organization’s data sustainability strategy.
But being energy efficient is not enough, bringing us to the issue of carbon emissions. Introducing a strategy to reduce their organization’s carbon footprint in data centers (i.e., GHG emissions) as well as that of third-party service providers is becoming critical for IT leaders not only from a corporate social responsibility perspective but also to cut costs and improve operational agility.
The three elements of a low carbon data center include:
- A good renewable energy strategy, and/or a location in a cool climate where free cooling is available, and/or on an electricity grid that has a low emissions factor
- The implementation of circular economy practices that manage the full life cycle of the IT assets to ensure an end-to-end approach is taken to extend the life of the assets and that, overall, reduce their environmental footprint
- Choosing service providers and vendors that offer energy efficient platforms, have demonstrated good sustainability practices and performance
Optimize data movement.
Moving data around over a network and in/out of the cloud can potentially consume a lot of energy and come with a significant carbon footprint where volumes are high. Reduce the storage and processing requirements by removing physical data flows that are no longer required and by shifting from point-to-point to hub-oriented integration patterns. Adopt more modern data integration techniques, such as data virtualization, which do not require data movement. By building toward data architectures that keep data close to the point of need and leveraging distributed and connected paradigms, organizations can reduce environmental impacts.
Other Principles
- Avoid unnecessary data duplication through common data repositories and modern integration approaches, such as data virtualization.
- Minimize the amount of data processed to achieve analytic goals by identifying where more “surgical,” or precise and lower volume, data access can meet business needs, for example.
- Understand the purpose and performance needs of the data, such as operational or archive data, or acceptable data latency, and don’t over or under deliver at the expense of resilience, quality, performance, regulatory requirements and more.
- Store data on the most passive storage medium suitable to its purpose via a tiered approach.