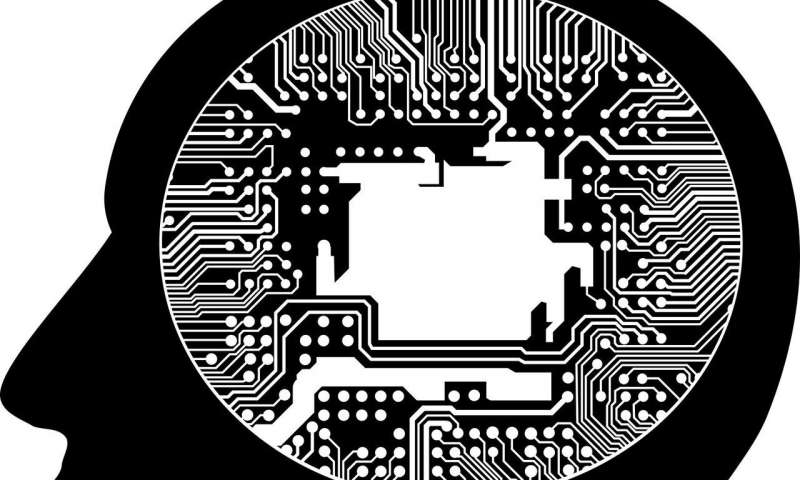
JULY 30, 2021 by Tanya Petersen, Ecole Polytechnique Federale de Lausanne
Collected at: https://techxplore.com/news/2021-07-machine-science.html?utm_source=nwletter&utm_medium=email&utm_campaign=daily-nwletter
Machine learning has burst onto the scene in the past two decades and will be a defining technology of the future. It is transforming large sectors of society, including healthcare, education, transport, and food and industrial production, as well as having an enormous impact on science and research.https://396b2166041191d2187646aafaf66bef.safeframe.googlesyndication.com/safeframe/1-0-38/html/container.html
A subset of artificial intelligence, machine learning is a process that helps computers to learn without direct instruction, and from experience. It does this by using algorithms to identify patterns within data, which are then used to create models that can make predictions. And data is the key. Machine learning, and the spiraling availability of vast amounts of data, promises to revolutionize the production of knowledge. Indeed, today’s exponential and virtuous cycle of growth in deep learning, among other technologies, has been compared to the Cambrian Explosion of half a billion years ago when life on Earth experienced a short period of very rapid diversification.
Professor James Larus, Dean of EPFL’s School of Computer and Communications Sciences (IC), agrees that machine learning and AI will have a profound impact on how we live and we have yet to see anywhere near its full potential.
“To me, machine learning is a very powerful tool that is still very much in its infancy and it is still somewhat of a ‘dark art’. We teach classes in machine learning, the underlying math behind it and are able to give students examples as to how it has been applied in the past, but we can’t give them principles because we literally don’t even know why it works as well as it does.”
EPFL’s Lenka Zdeborová is working on this fundamental question. Associate Professor ofPhysics, Computer Science and Communication Systems in the Statistical Physics of Computation Laboratory—part of the School of Basic Sciences (BS) and IC—she is passionate about advancing the theory of what is computable and what’s possible with machine learning and artificial intelligence.
“In sciences we want to understand the objects we study better, the objective is not fixed. We need to come up with the objective so that the machine learning system is useful in the scientific endeavor and look at the role that machine learning is playing in changing the very scientific method. It’s a fascinating field that has emerged as machine learning has become very successful in the past decade.”
With colleagues from physics, chemistry, engineering and life sciences, Zdeborová has just launched a new doctoral course lecture series on scientific machine learning that will explore the latest work being undertaken at EPFL and globally.
Another EPFL initiative—the Machine Learning 4 Science project component of the Machine Learning course of IC Professors Martin Jaggi and Nicolas Flammarion—is building cross campus collaborations, matching science projects from laboratories across all disciplines with students who will bring their machine learning expertise to new fields. Between 2018 and 2020 more than 600 students participated in projects proposed by 77 labs across EPFL, and even outside institutions including CERN.
“It’s the largest masters level course on campus and students across all disciplines want to learn this tool as they know it will be useful to their future careers. They can go to any lab on campus and do a hands-on project, collaboratively in an interdisciplinary way. It’s a real win-win and I think it’s fair to say that both sides feel that they benefit from the structure,” says Jaggi.
One of the projects in the last round originating from Cathrin Brisken’s lab in the School of Life Sciences (SV) related to a machine learning algorithm to distinguish mouse cells from human ones, particularly useful for cancer research. Oncologists typically study tumors by grafting human cells onto mice, but then the problem is telling the two kinds of cells apart. That usually involves several rounds of fluorescence staining and analyzing many tissue samples before finding the human cells. However, IC student, Quentin Juppet’s program simplifies all that by automating the cell-classification process. It is so promising that he turned it into a master’s thesis with the results recently published in the Journal of Mammary Gland Biology and Neoplasia.
Another, also originating in the School of Life Sciences, involved using machine learning to categorize mutant phenotypes from images of zebrafish embryos. Professor Andrew Oates is Dean of the School and head of the Timing, Oscillations, Pattern Laboratory. “My lab has participated twice and each time we have engaged with a really special group of students who have shown initiative and creativity in addressing a real scientific problem in the lab using machine learning. As far as I know this project is a first in the field of embryology with implications for the more efficient use of zebrafish as a system to model human genetic disorders. We would not have attempted this work if we didn’t have the chance to join up with the Machine Learning 4 Science program,” he says.
Other work looked at an incredibly diverse set of research questions: predicting stroke severity using pacman game data; the automatic detection of available area for rooftop solar panel installations; avalanche forecasting; music beyond major and minor; and, improving freshwater quality measurements.
For James Larus the future is here and it will only get more amazing, “Currently, machine learning is based on a model developed in the 1940s of how the brain works, and it wasn’t even accurate at the time. Now we are exploring brain inspired machine learning, guided by the latest neuroscience, to develop more sophisticated and effective models and to build next generation artificial intelligence systems. So, I’m really hopeful that there will be a long period of progress in machine learning and a huge expansion in successful applications. It will change science forever.”
More information: Quentin Juppet et al, Deep Learning Enables Individual Xenograft Cell Classification in Histological Images by Analysis of Contextual Features, Journal of Mammary Gland Biology and Neoplasia (2021). DOI: 10.1007/s10911-021-09485-4
Provided by Ecole Polytechnique Federale de Lausanne