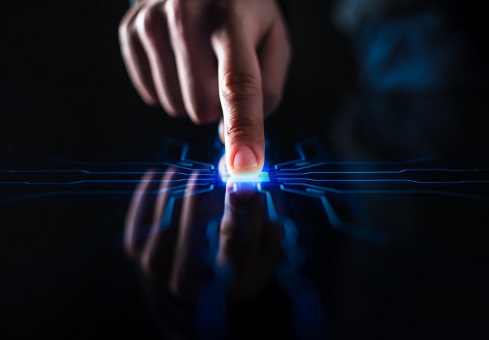
4/20/2021 by Lisa Morgan
Collected at: https://www.informationweek.com/big-data/ai-machine-learning/10-things-your-artificial-intelligence-initiative-needs-to-succeed/d/d-id/1340729?_mc=NL_IWK_EDT_IWK_daily_20210420&cid=NL_IWK_EDT_IWK_daily_20210420&elq_mid=103384&elq_cid=27653255
In the race to implement AI, some companies may overlook important details that can mean the difference between success and failure.
The rush is on to implement in a battle for competitive advantage. However, in the haste to implement, some organizations are stumbling because their initiative lacks a solid foundation.
“People want to solve problems with AI just because it’s AI and not because it’s the best solution,” said Scott Zoldi, chief analytics officer at analytics decisioning platform provider FICO. “It has to be soup to nuts. How are we going to develop AI from a governed perspective of having a governance process that talks about the data, the success criteria and the risks from both a project perspective and an ethical perspective?”
Some AI initiatives falter because the thinking that went into them was inadequate. For example: ADVERTISING
- The AI initiative is created separately from the business strategy so it fails to make a strategic impact.
- The success criteria are overly broad because they fail to include a success metric (E.g., “We want to be more competitive” as opposed to “We want to reduce fraud by 15% while reducing the number of false positives by 30%.”)
- The change management aspect wasn’t considered so the initiative faces resistance.
“Shared capabilities or shared data across business units is becoming more important than the autonomy of individual units,” said Marco Iansiti, David Sarnoff professor of business administration at Harvard Business School, who heads the technology and operations management unit and chairs the Digital Initiative. “This causes all kinds of difficulties in traditional organizations because all of a sudden, you have a person who runs investment banking that has never shared anything with the person who runs wealth management. And all of a sudden, they are both interested in leveraging some of the same algorithms and some of the same components. They have to standardize because before they didn’t have to.”
Making sure security teams have adequate resources to invest in frameworks like zero trust and are nuanced in the latest attack methods and vectors will help ensure systems are adequately monitored to thwart potential threats.Brought to you by LogRhythm, Inc.
The use of AI has become such a strategic issue that CEOs are getting involved in defining what their company’s AI strategy would look like.
“Earlier, we were seeing it was the CIO, CTO and some CXOs, but now the leading CEOs realize that this is going to redefine the future of their industry and the future of their own company,” said Arnab Chakraborty, global managing director, applied intelligence North America lead at global consulting company Accenture. “They’re looking at this as a reinvention of their business in the context of where things are headed with AI.”
Some of the common missteps can be avoided or minimized by thinking through the initiative in a holistic manner and involving those in the value stream who can help think through the various aspects — opportunities, risks, potential impacts, success factors, data requirements, compliance issues, governance, etc. Other success factors follow.
Be Clear About Why You Need AI
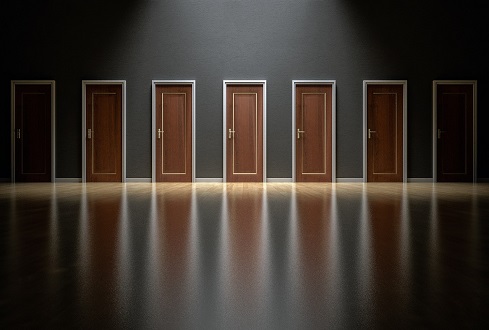
Many organizations are under competitive pressure to adopt AI. However, a better approach is to step back, take a look at what the organization wants to accomplish and then consider what’s actually needed to do it.
“Make sure you really need AI. Creating an AI or machine learning algorithm without a plan for how to use it and managing the affects you expect from it is a waste of money and talent. Scoping the problem is the first step,” said Theresa Kushner, senior director of data intelligence and automation global IT consulting company NTT DATA Services. “My experience has shown that approximately one in six projects ever makes to a return on investment.”ADVERTISING
Bear in mind AI is used for different purposes such as cost reduction, increasing revenue, predicting an outcome or optimizing a process. Even if you’ve concluded AI could help with such a problem, you may lack the data you need to solve the problem, Kushner said.
Train on Good Quality Data
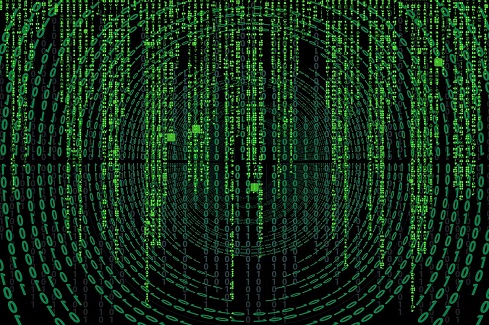
Never underestimate the power of data. If it’s dirty, which is its natural state, it’s inconsistent, inaccurate, incomplete or duplicative. When dirty data is used as training data, bad outcomes can result such as poor recommendations and faulty conclusions.
“AI has tremendous power, but any AI solution is only as good as its source data. Before any AI implementation, steps must be taken to ensure data quality and availability, as well as to define clear and measurable KPIs,” said Arthur Iinuma, president of mobile and web app platform provider ISBX. “Comprehensive, unpolluted datasets are vital to ensure the best results.”
Realize That Lab Results and Real-World Results May Differ
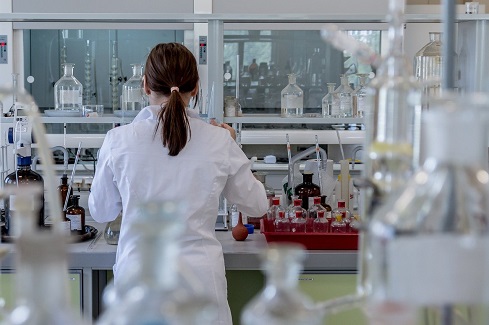
Some AI pilots work well in a lab but not in the real world because the real world is far more complex and random. Similarly, one successful use case is not a guarantee that the AI will perform as well when applied to another use case.
“Real world AI is not entirely different than AI in the lab, but the solutions should be more complete, stable and adaptable,” said Rotem Alaluf, CEO of AI company BeyondMinds. “Like the difference between Roger Federer and a child playing tennis — same game, same rules but different skills and ability to react and adapt to surprises. We need to understand the limitations of lab AI, learn what is needed to create value from it in the real world and employ this in a scalable way within an organization.”
It’s a Team Effort
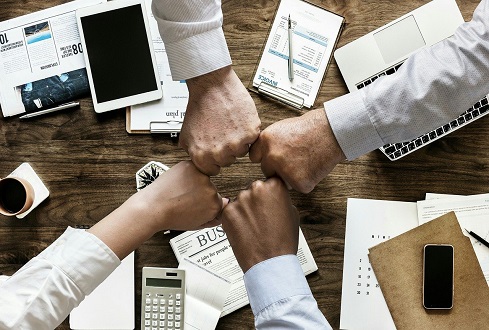
AI and data scientists seem to go together. However, AI is actually a team sport. It needs executive sponsorship and cross-functional collaboration.
“Getting relevant business and product decision makers, data owners and managers, engineering teams and data scientists ‘on the same team’ is crucial. If one of these stakeholders isn’t brought in, there is a slim chance of success,” said Betsy Hilliard, principal data scientist at data science consulting firm Valkyrie. “In large organizations, especially ones with heavy divisions between business functions, building the needed cross-functional team can be difficult. Make sure you have the support in the reporting chains of each area.”
For example, if the data science team sits in a different part of the organization than the product team leading the AI initiative, it’s wise to get the support from the leadership above the data scientists to avoid prioritization or resourcing conflicts, Hilliard said.
Align the AI Initiative With the Product Roadmap
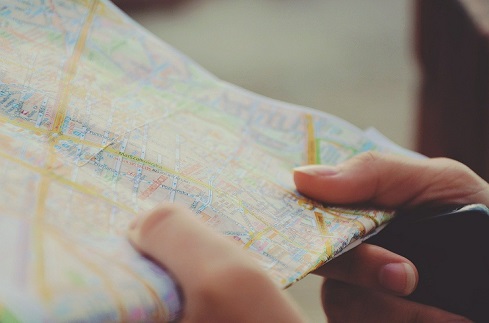
An AI initiative for its own sake is not an AI strategy, nor is hiring a data scientist. John Langton, director of data science at global professional information, software solutions, and services provider Wolters Kluwer said teams must understand that AI is not the product, it is an enabler of new products. However, product managers tend not to have a good grasp of what is and is not possible to do with AI.
“A successful AI initiative needs to center on ongoing dialogue between product development teams, leadership and tech leadership to develop sound AI tools. Good data scientists can educate product teams on the art of what is possible around the technology while product teams can bring the market and customer expertise to make sure the actual problem is solved,” said Langton. “This also allows both groups to incorporate AI checkpoints into product roadmaps without treating it as a separate R&D product. Directly connecting data scientists and product teams allows you to set expectations about what AI looks like once it has been applied.”
Monitor Models for Drift
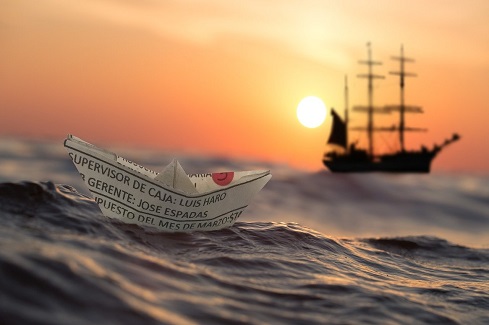
As new data comes in, models tend to drift, becoming less accurate over time so they may need to be tuned or retrained.
“To build successful AI initiatives, IT teams must embrace the dynamic nature of AI models and invest time and energy to train them, similar to the way that a company veteran must train a new employee,” said Bob Friday, VP and CTO for the AI-driven enterprise at networking and cybersecurity solution provider Juniper Networks. “As part of the process, enterprises must have experienced technology teams in place to analyze the performance of and results provided by AI models. By providing constant feedback, AI models will adjust their logic and in turn, solve problems more accurately and efficiently.
Ethical/Unethical AI Can Impact Your Company’s Brand and Reputation
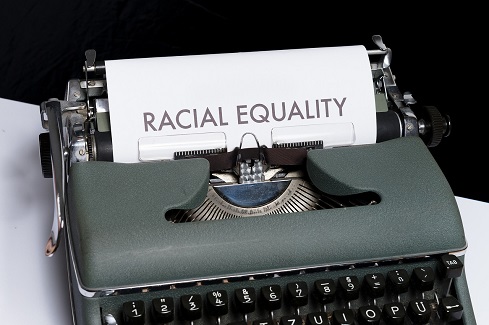
AI gone awry can cause all kinds of problems including legal issues, regulatory fines and reputational damage. Several years after Microsoft’s Tay bot fiasco and Amazon’s sexist HR bot gaffe, these dramatic examples are still being used as examples of what can go wrong years after the fact if the AI isn’t closely monitored (Tay bot) or the training data is biased (HR bot).
“AI will make decisions about all sorts of things, but is it making good decisions? More often than not, it’s fraught with unconscious bias from ‘dirty’ data resulting from humans,” said Alex Spinelli, CTO of LivePerson. “I strongly believe that it’s not enough for AI to help us be smarter, faster, more productive, what have you. It needs to be a force for good in the world. Companies [which] view that as Pollyanna and not sound business strategy might very well find themselves addressing legal issues in the future, not to mention having ethics is an amazing recruitment tool and enhances employee satisfaction.”
While AI Is Learning, People Should Be Learning, Too
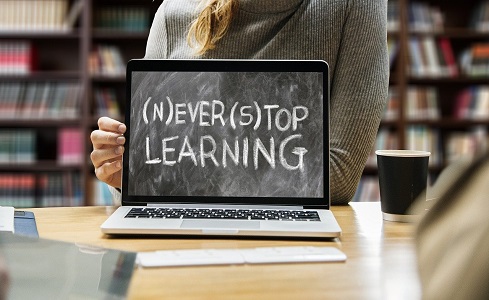
Today’s working professionals are told they need to become lifelong learners if they want to be successful. Meanwhile, AI systems are “learning” how to do all kinds of things whether that’s recommending a new movie to a customer or identifying suspicious behavior in a subway during rush hour. As AI augments humans at work, helping them do their jobs more efficiently, both should be learning simultaneously. The human learns how to use the AI more effectively over time while learns the users’ preferences and behavior thus learning how to work with the human more effectively over time. Both may also need ongoing training so they can adapt more effectively to change.
“One of the reasons why some initiatives fail to provide ROI is the skill gap, or lack of training in personnel after a company’s tools and processes have been updated, upgraded and upskilled to include AI,” said Anthony Ciarlo, VP alliance relationships at multinational professional services network Deloitte. “AI is ever-changing and it takes an organizational commitment to invest in your personnel and to invest in a mantra of career-long or life-long learning!”
Avoid “Big Bang” Implementations
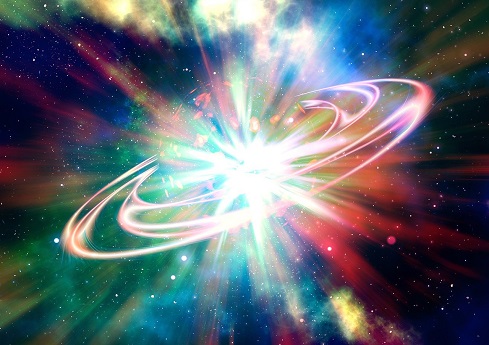
Successful AI initiatives happen by design, not default. That said, it’s entirely possible to try to address too much too soon before learnings can be incorporated, resulting in lackluster outcomes and low or no ROI.
“One thing enterprises should do to succeed in their AI initiatives is to adopt AI incrementally. After identifying an AI/ML use case, it has to be implemented in an incremental way as the desired outcome may not be achieved in the initial deployment,” said Chida Sadayappan, cloud AI/ML leader at Deloitte. “The collection and preparation of data to be modeled for an AI/ML has to go through an iterative process even after initial deployment. Thus, approaching the AI initiative in an incremental fashion tends to be the success factor.”
AI Is More Than Algorithms and Models
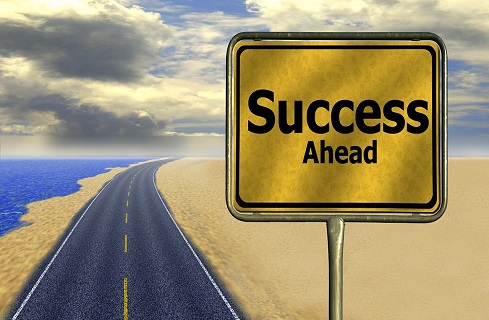
AI is often viewed in only technical terms (e.g., models and algorithms) when its benefits and successes also depends on people and processes. The purpose of AI should be to advance business objectives.
“Start by clearly defining the intent of the AI project and then define specific use cases for the technology that will help determine what types of AI solutions are needed and how they will be integrated into your infrastructure,” said Seth Dobrin, global chief AI officer at multinational technology company IBM. “From there, evaluate the data sources feeding into the AI model and set concrete actions for the AI by using statements of intents as a guide for the technical implementation. Through this process, businesses can operationalize AI throughout the business by connecting every solution into a singular AI strategy.”